Synthetic Test Data Vs Masking
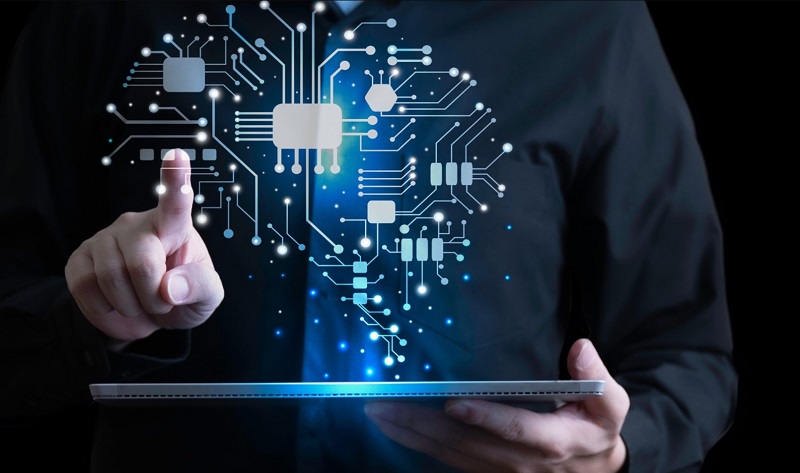
Because of the enormous amount of users’ personal data coming into the digital world every day, there is a need for data privacy, cybersecurity, and the use of personally identifiable information for the purpose of systems development and testing. The General Data Protection Regulation (GDPR) has asserted the need for masking and synthetic test data for the protection of personal data. The need for test data is increasing more than ever, however the Quality Engineering (QE) practitioners are struggling to get access to the sources of anonymous data. To comply with GDPR, anonymous data can be produced under synthetic data or mask production data. This article compares synthetic test data with masked data.
Although even now some businesses conduct testing using modified production data to conceal identifiable personal customer information, such as names, contact details, account details, etc. The other alternative of production data is the manual generation of data, which takes considerable resources and time. Test Data Management tools have allowed us to mask data obtained from production, or generate synthetic test data.
From selecting the source of data to encrypting it, from data production to synthetic data generation- test strategies differ with a test environment because of the varied sources of EDI test data. For example, an insurance company has a huge masked data bank, along with a golden key containing data combinations obtained from production and masking. In case, test data is not available, a test data portal will generate synthetic tests using TDM tools. You must know the upside and the downside of both approaches because it will help you in deciding the percentage of production data and synthetic data.
The common practice for using anonymous data is through creating referential data, sourced by masking. This allows the generation of anonymous data by obtaining personally identifiable information (PII) and then masking it to make it anonymous.
According to GDPR, the technology captures much more than basic personal information like names and addresses. Therefore, PII must be anonymized, and if new fields are to be concealed, then even the implemented solutions must be revalidated.
Synthetic Data
On the contrary synthetic test data saves you from the trouble of masking and anonymity, by offering a more practical solution. When the data is generated within the test region, no data privacy is being violated and there is no need to take extra steps for complying with regulatory needs. TDM tools can help with synthetic data generation, which is equally good as real-time data. The only time when an enterprise needs to have real data, and can’t rely on synthetic data, is when the inherent complexity of data is required for aggressive testing, and only real data is ‘fit for purpose data. The fit for purpose data should have the following traits:
Referentially intact data:
This includes referentially intact data which has to be present in records. In the case of synthetic transactions, there must be corresponding synthetic information about customers in customer records, and product information in product tables.
- Production Scenarios
Synthetic data must also contain detailed production-like business scenarios. In case of the absence of correct combinations, data testing cannot be conducted thoroughly and properly. This can lead to data inconsistencies and can delay the QE cycle.
- Consistent with business processing
Synthetic test data must be consistent with the pattern data that is handled, managed, processed, and manipulated during an end-to-end flow.
Conclusion
Although generating synthetic data for testing is easier that masking real data for anonymity, however, it has other complications like consistent and integrated data for strong strategy, good business, and application knowledge. It requires dedicated effort to create comprehensive fir-for-purpose synthetic data.
While both masking and synthetic data have their own bright sides and downsides, some testing teams adopt a hybrid strategy of generating test data using functional automation. This is possible by generating synthetic data in a test environment without any knowledge about data sources, and combining it with only valid business combinations.
Hybrid test data generation is one of the efficient and effective test data generation strategies because it ensures data privacy and security, and automation which expedites the data generation process. However, a hybrid solution cannot be implemented in all cases. You must choose your test data strategy as per your business requirement. To know more about possible test data strategies talk to our representative at GenRocket.